DANNCE
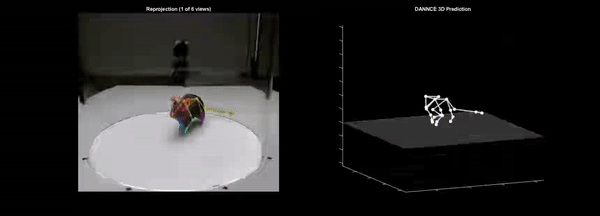
DANNCE is a direct method for 3D markerless pose estimation and tracking developed by Timothy Dunn, Jesse Marshall, and colleagues in Bence Ölveczky’s lab. It works on recorded video data from multiple cameras. The key innovation in this method is to use geometrical methods to create 3D feature spaces in which shared features across cameras are used to track animals and infer pose information. This is done by extending 2D confidence maps to three dimensions through the use of volumetric convolutions. Images from each camera are projected onto a common volume space. A 3D convolutional neural network is then used to provide a single confidence map for the pose data over the 3D space. The method was demonstrated to work for data from individual or groups of behaving rodents, non-human primates, and birds
This research tool was created by your colleagues. Please acknowledge the Principal Investigator, cite the article in which the tool was described, and include an RRID in the Materials and Methods of your future publications. RRID: SCR_023061
Documentation
Read the Nature Methods paper!
See Also:
The DANNCE of the rats: a new toolkit for 3D tracking of animal behavior. Karashchuk P, Tuthill JC, Brunton BW.
GitHub
Get started with the necessary files and check out the tutorials from the source!
Check out projects similar to this!