KineWheelArena-DLC
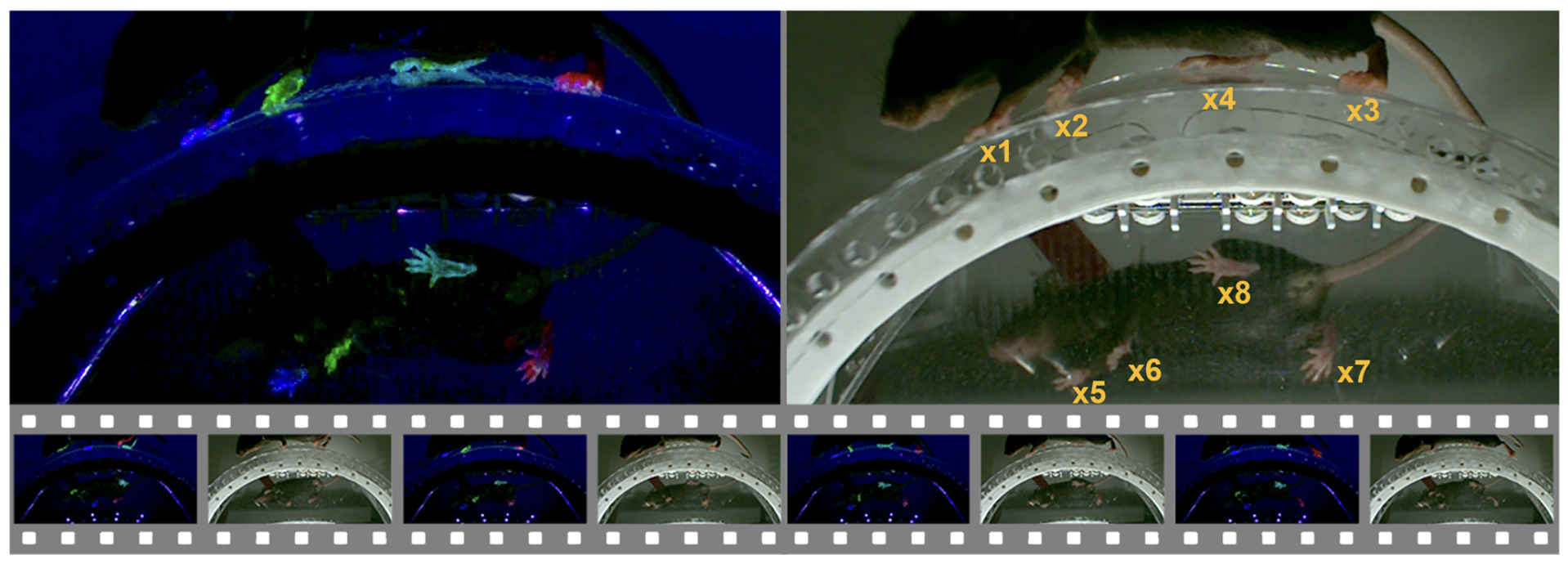
Björn Albrecht and colleagues in the lab of York Winter from Humboldt Universität have developed an innovative system that enhances the efficiency of markerless pose estimation. Their method integrates existing technologies like KineWheel and DeepLabCut, specifically addressing one of the most time-consuming steps: the manual labeling of training frames.
The system’s design alternates between two types of illumination, standard white light and ultraviolet (UV) light, at a high frame rate. As mice move on a specialized transparent wheel, their paws (treated with safe fluorescent dye) briefly fluoresce under UV flashes. These illuminated frames reveal precise paw positions, which the system uses to label corresponding white-light frames through a Python script. Early testing of this toolkit add-on demonstrates that the high accuracy expected from markerless tracking is maintained, while drastically reducing manual labeling work.
This research tool was created by your colleagues. Please acknowledge the Principal Investigator, cite the article in which the tool was described, and include an RRID in the Materials and Methods of your future publications. RRID is currently pending, stay tuned!
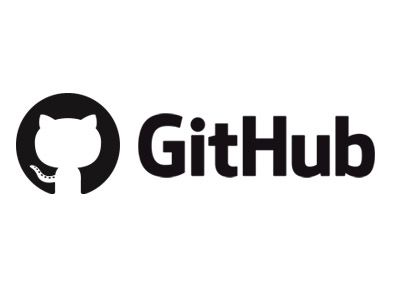
Access the code!
All design files are available in a GitHub repository.
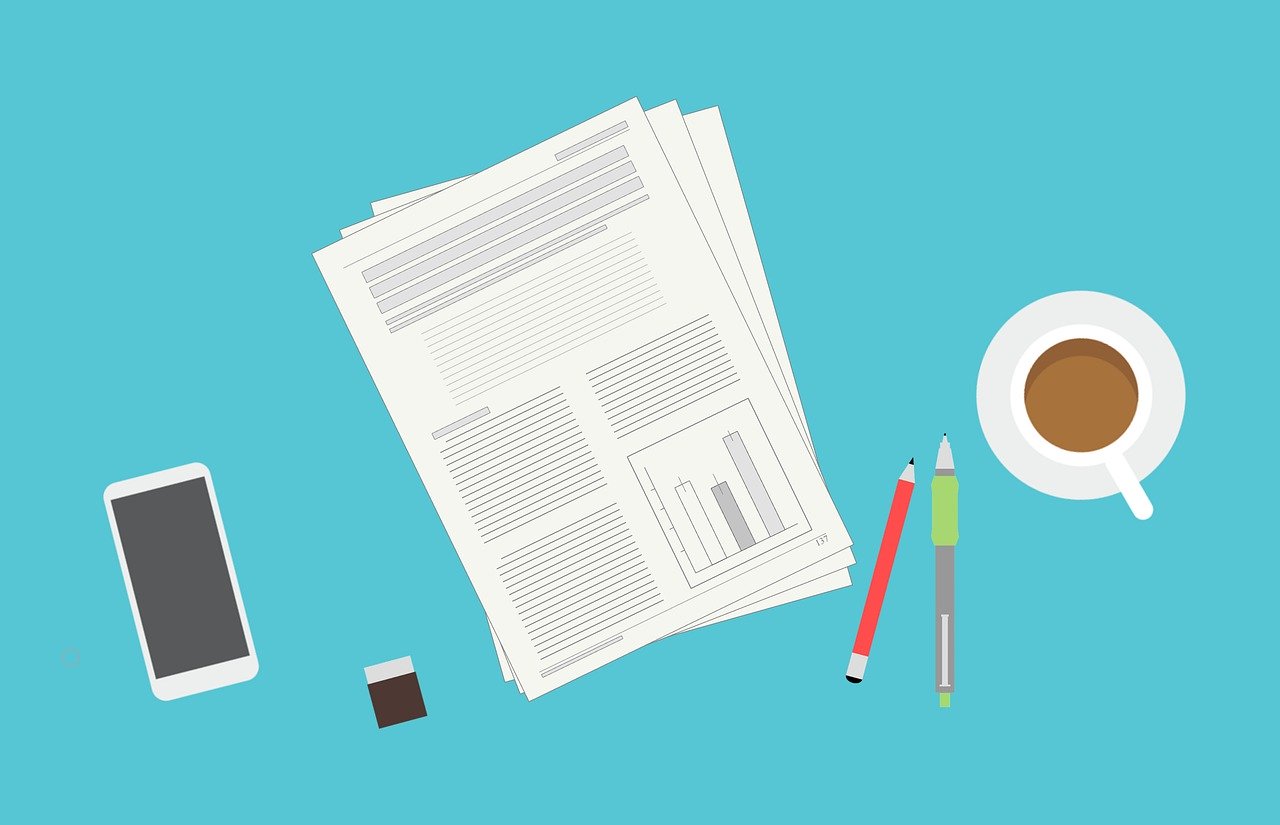