SaLSa
Dr. Shuzo Sakata, from the University of Strathclyde, has developed a new tool for classifying animal behaviors. This MATLAB package, called SaLSa, offers an innovative approach to analyzing and categorizing behaviors using pose estimation data. Traditional manual annotation of behavioral events is time-intensive and subjective, making automated and scalable alternatives a big necessity. SaLSa integrates semi-automatic labeling with machine learning, specifically Long Short-Term Memory (LSTM) neural networks, to classify behavioral syllables (distinct, repeatable behaviors).
The process begins with semi-automatic labeling, where key behavioral features are identified using pose data, reducing manual efforts. These initial labels serve as input for the LSTM to classify and predict behavioral events. The LSTM learns from the temporal patterns in the labeled pose data, enabling the high-precision detection of simple and complex behaviors.
SaLSa demonstrates versatility by accommodating a wide range of behavioral datasets and producing results comparable to or exceeding fully manual annotations in accuracy and consistency. Additionally, the semi-automatic step ensures that domain knowledge is incorporated into the training data, enhancing model reliability. The method facilitates large-scale behavioral studies, offering new avenues for analyzing animal decision-making, motor control, and social interactions.
This research tool was created by your colleagues. Please acknowledge the Principal Investigator, cite the article in which the tool was described, and include an RRID in the Materials and Methods of your future publications. RRID:SCR_026182
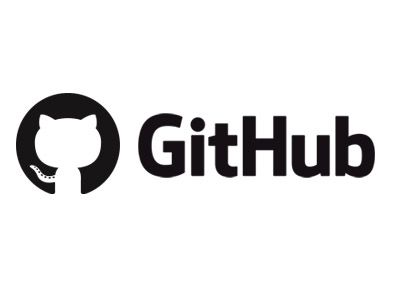
Access the code from GitHub!
Check out the repository on GitHub.
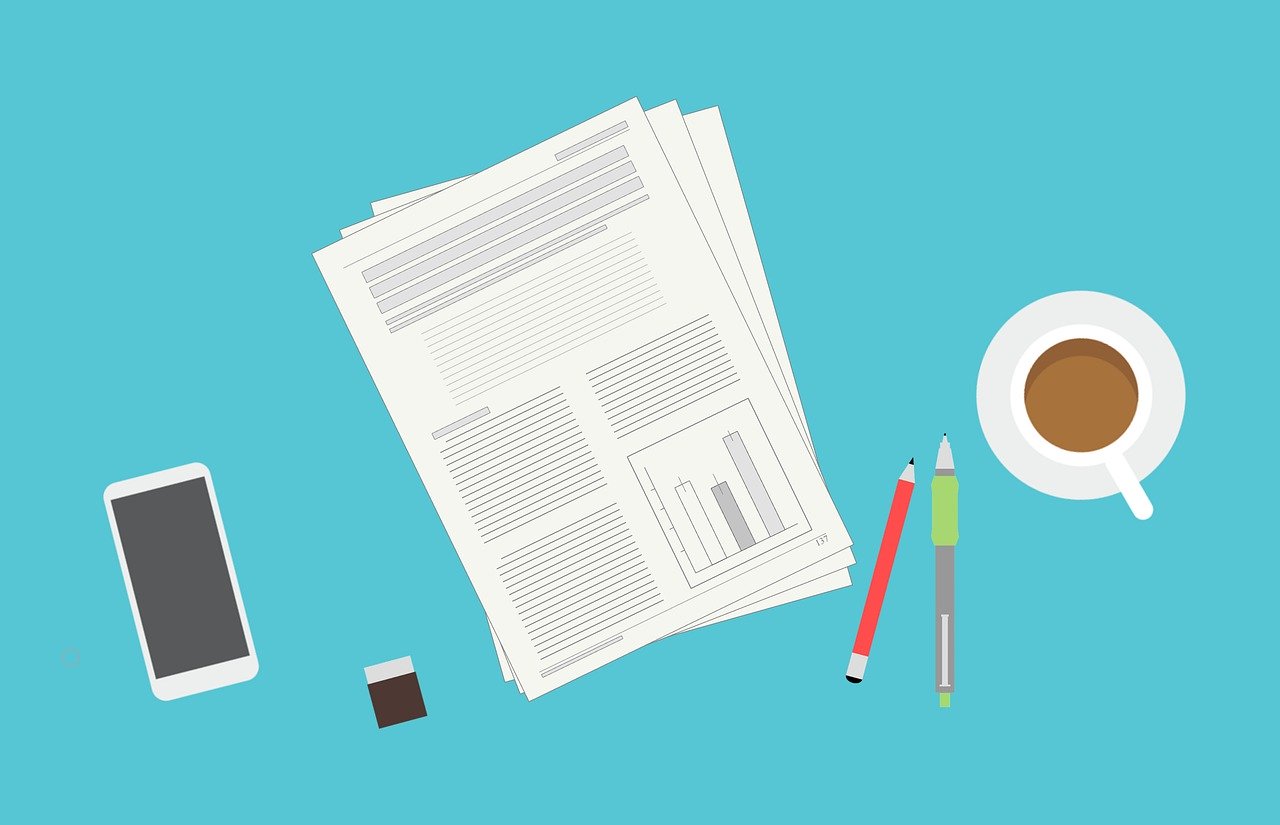
Read more about it!
Find out more on SaLSa in their eNeuro publication!
Check out projects similar to this!